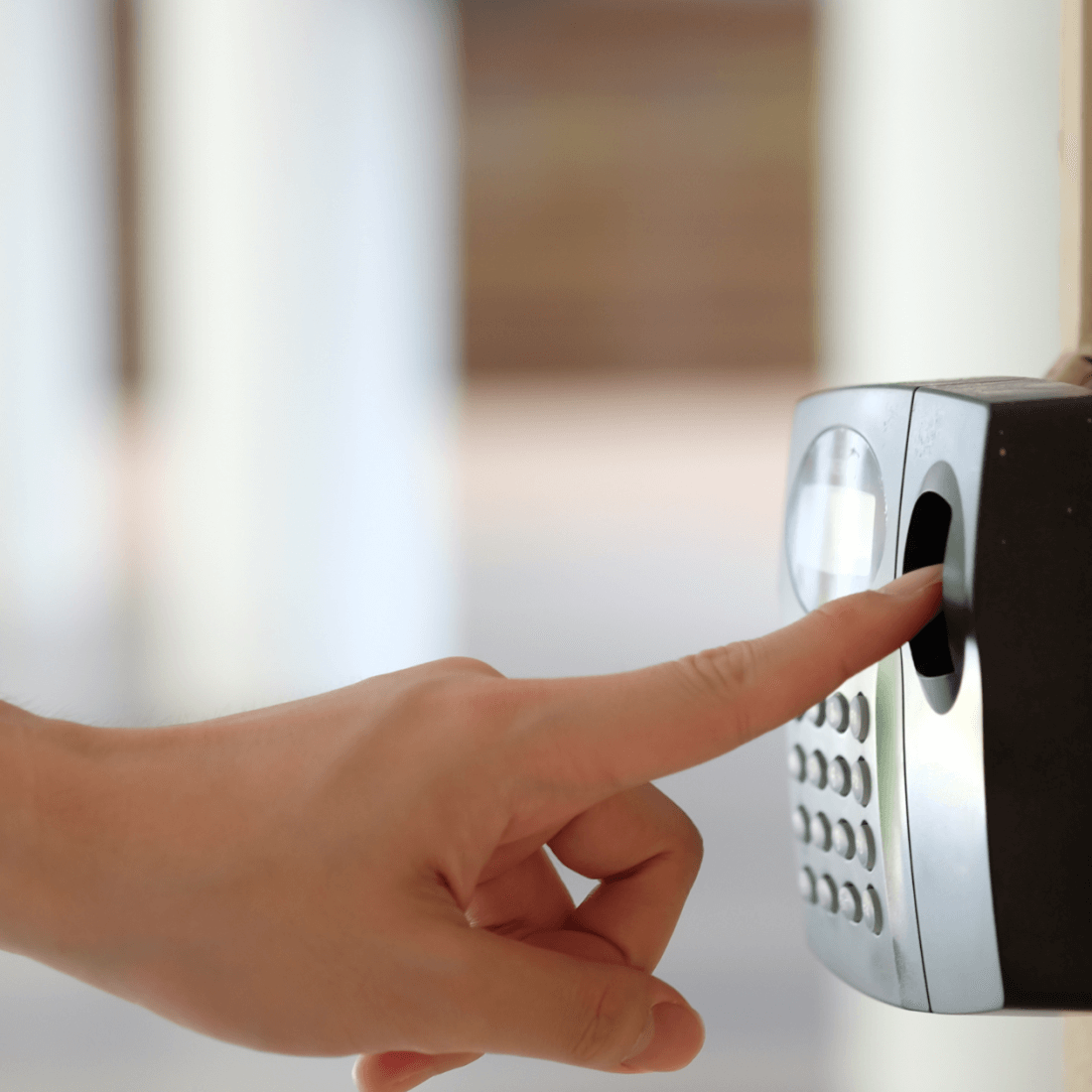
In this day and age of artificial intelligence, machine learning, and big data analytics, the majority of manual labor has already been replaced by sophisticated computers. From big conglomerates now machine learning is also seeping into small and regular-sized businesses by helping them automate various repetitive or regular tasks such as tracking attendance.Ā
Application of Machine Learning Algorithms in Biometric Attendance Systems
We already know that ‘biometric technology’ is a real-time system that confirms an individual’s identity by employing distinctive features such as fingerprint patterns and facial geometrics. Let us look at how the combination of machine learning and biometric technologies has transformed the tech sector.
Machine learning makes extensive use of structured data in order to generate findings or make predictions based on the data provided. Machine learning is currently being applied in Google search algorithms, spam mail filters, Facebook friend recommendations, and online buying recommendations.
Machine learning approaches assist in the identification, recognition, and classification functions required for the creation of biometric systems.
Key Advantages of the Fusion:
Accuracy and Security:Ā
Traditional methods often suffered from inaccuracies due to manual errors or fraudulent practices. Machine learning algorithms, when trained on extensive datasets, can accurately differentiate between genuine and manipulated biometric data, ensuring the security of attendance records.
Adaptability:Ā
Machine learning algorithms can adapt to changing conditions and variations in biometric data. This ability to accommodate differences, such as changes in facial hair or voice tone, makes the system more robust and reliable. It can help with face recognition systems and even fingerprint readers.
Scalability:Ā
Biometric attendance systems powered by machine learning can be seamlessly integrated into larger organizational frameworks. This makes them suitable for organizations of varying sizes, from small businesses to multinational corporations.
Analytics and Insights:Ā
Machine learning algorithms can also derive insights from attendance data. By analyzing attendance patterns, organizations can identify trends, optimize resource allocation, and make informed decisions.
Anomaly Detection:Ā
ML algorithms can detect unusual patterns in attendance data, such as duplicate entries or suspicious activity. This adds an extra layer of security to attendance management.
Challenges and Considerations:
While the benefits are clear, the convergence of machine learning and biometric systems also presents challenges:
Privacy Concerns:Ā
Collecting and storing biometric data raises privacy concerns. Organizations must ensure strict data protection and compliance with regulations.
Technical Challenges: Implementing and maintaining ML-powered systems requires technical expertise, infrastructure, and resources.
Data Security:Ā
Biometric data, being uniquely tied to individuals, poses significant security risks if mishandled. Robust encryption, access controls, and compliance with data protection regulations are paramount to thwart breaches and identity theft. Safeguarding this sensitive data ensures trust, mitigates potential harm, and upholds the integrity of biometric attendance systems.
The combination of machine learning and biometric attendance systems marks a significant leap forward in attendance management. Organizations that embrace these technologies stand to benefit from enhanced accuracy, security, and efficiency. However, it’s crucial to address privacy concerns, invest in technical capabilities, and ensure data security to fully leverage the potential of this revolutionary approach to attendance tracking.